Artificial Intelligence (AI) and its latest evolution Generative AI (GenAI) are not only the hottest buzzwords in technology, but also likely the most transformational value drivers for businesses and people. We felt the same way for technology evolutions in mobile, social and cloud, which are now mainstream in society and the workplace. Hence, every business needs a strategy to leverage GenAI as an offensive or defensive measure to stay relevant and competitive. We all have seen the demise of companies that have failed to stay current with technology.
Data is at the heart of GenAI
The core premise of GenAI systems is to digest vast amounts of unstructured data, train models and use them to find connections, learn, build on prior knowledge and synthesize it into usable forms. The system becomes smarter as it learns more and with feedback, similar to humans becoming more intelligent with education and experience. The most relevant category GenAI models for most enterprises are Large Language Models (LLMs) due to their ability to synthesize vast amounts of diverse datasets and accept natural language as inputs and produce natural language outputs. But where does this data come from? The key is to harness the power of valuable data that may be housed in a wide range of systems, from legacy to new.
Cloud makes GenAI reachable for any business
In its early innings, companies needed a vast amount of technical talent and computational resources to leverage GenAI. You had to be a Tesla or Google to venture into this territory. However, now with the advent of OpenAI and followed by several such initiatives, nearly all public cloud providers have solutions available to anyone requiring only modest effort and cost.
Data privacy issues can become challenging with GenAI
Anyone can now have an OpenAI account and use ChatGPT to answer questions, organize information and create content. This works well to plan a weeklong holiday itinerary at your favorite destination or to check your code for a computer science assignment at school. However, when employees at businesses start feeding proprietary data into GenAI interfaces, it bleeds into the public domain and innocently creates competitive and privacy issues. Would you want your competitor to learn from you to beat you at your game?
What’s your strategy to bring GenAI to your business?
To protect proprietary data and trade secrets while leveraging the power of GenAI, it becomes critical for businesses to have a thoughtful AI strategy. It always starts with a simple question: Why? Followed by another one: How?
Knowing what you want to achieve from GenAI is the most critical “Why?” question. At Skytap, we have seen several use cases from our customers:
- Augmenting internal knowledge bases to find relevant answers
- Improving productivity for developers, content writers, marketers, etc.
- Improving customer experience for support, customer service, etc.
The next question is “How?” For business use, it typically requires your own private instance of GenAI LLM and a cognitive interface that you can source from a cloud provider or deploy on your own.
Problems of bringing data to GenAI
These technologies are empty shells for all practical purposes unless you feed them with your data to align with your “Why?” question. Unfortunately, that’s where the problem lies. Most data in enterprise is distributed across multiple systems including legacy ERP and databases that run on architectures that are not amenable to run in cloud infrastructure. Hence you can try to extract data from your distributed on-premises systems and feed it into centralized cloud-based GenAI engines, but the cost and complexity can balloon quickly. Alternatively, you can run these AI engines on-premises, but the problems of bringing the data together remain the same.
Bringing systems, not just data, to the cloud
Hence for an effective GenAI strategy, businesses need to bring their systems and applications to the cloud, not just the data. This is easier done for modern container-based, virtualized or packaged applications based on popular x86 based architectures. However, some legacy ERP or business-critical applications based on RISC/Power architectures cannot be readily migrated or operated in cloud environments. Since these applications often host crown jewels of data for several industries including banking, finance, insurance, healthcare, retail, CPG, manufacturing, industrials, etc., the “How?” question may block these businesses from realizing their “Why?” to take full advantage of GenAI capabilities in their AI strategy.
What are the options for legacy specialized workloads?
Of course, you can rewrite these workloads for the cloud, but that takes time and resources. Not to mention the risk of business continuity when errors are introduced in such transitions of proven, long running systems. You can migrate all systems to a centralized hosting provider and connect to the cloud via Direct Connect or Express Route networking technologies. In this case, you cannot leverage the cloud operating model and these systems become another island in your cloud strategy posing compliance and security issues.
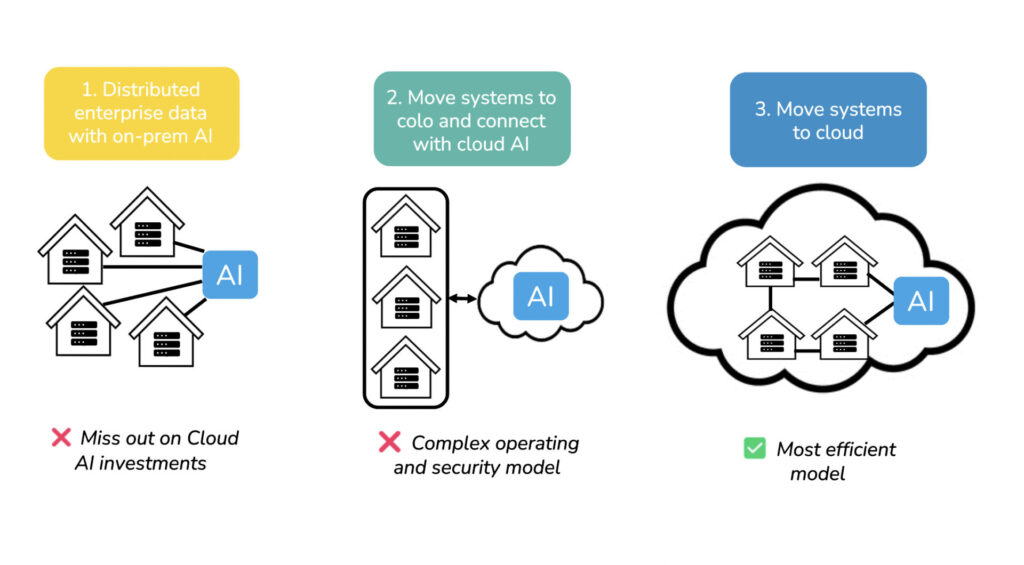
Multi-billion industrial conglomerate transforms with use of legacy data and AI
Faced with these challenges, a multibillion industrial conglomerate decided to partner with Microsoft Azure, OpenAI and Skytap to find the optimal solution. Its original “Why?” was to synthesize the data captured in its ERP systems located in its regional offices to improve business efficiency and effectiveness by running holistic analytics on sales trends, buying behaviors, technical decisions and customer experience. The enterprise later stretched to using this data for GenAI to provide a natural language based interface for its technical, commercial and sales teams to answer questions for installation, capacity planning, technical support, etc.
The ”How?” was to first migrate all of its legacy ERP systems running IBM i or AIX on Power hardware to Azure leveraging Skytap. Unlike traditional “lift and shift” approaches that need massive services investment, this was largely achieved via self service in a matter of days using Skytap’s cloud-based portal with reusable templates. Thereafter, just like any other public cloud service, the enterprise could focus on operating its applications while the Power, IBM i and AIX were provided as Cloud IaaS with a consumption-based commercial model.
Once its ERP systems were running in Azure, it became very easy to feed all the data into a data lake for analytics and vector databases for training AI models. It chose to use OpenAI LLM with private refinements leveraging its context and data to make it useful while protecting its data privacy. It used a private instance of ChatGPT to create an internal natural language interface to provide GenAI assistance to its business and technical teams. These approaches helped it increase its competitiveness, drove double-digit growth in an otherwise flat industry, and established the company as a thought leader in its industry.
Solution architecture
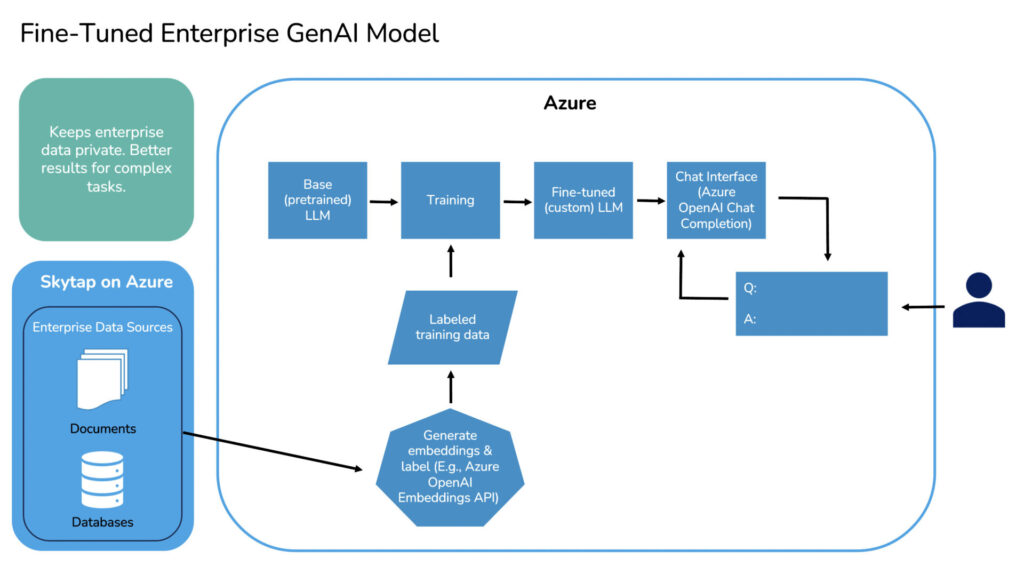
Other approaches
Enterprises that have more organized data in knowledge bases and do not have resources and talent to have their own fine tuned LLM can combine Azure’s Cognitive Vector Search capabilities with Azure Open AI services for Retrieval Augmented Generation (RAG) to return context sensitive results. This is less resource intensive and easier to implement although not as optimized as the fine-tuned LLM.
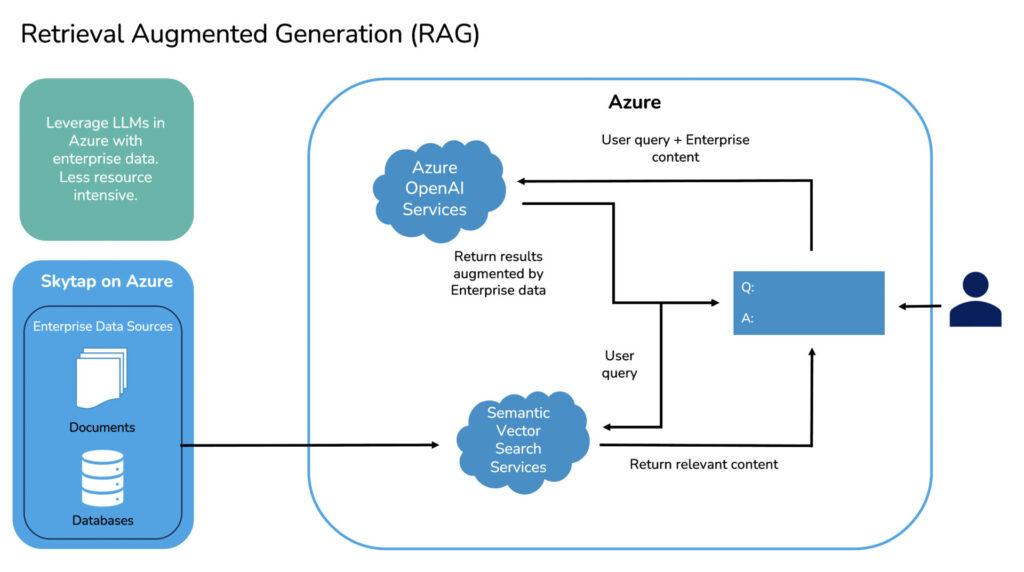
How to get started with AI?
To get your AI strategy on a sound footing, it is critical to answer the “Why?” question. If you can answer it without needing any data or capabilities in your legacy systems and are satisfied with the status quo in operating these systems, you can just ignore them. However, if your AI strategy cannot be complete without tackling these systems, there is an option to bring these systems to the cloud and bring the GenAI capabilities of the cloud to these systems through Skytap.
Looking forward to hearing about many more GenAI journeys!
Meet the author:
Rahul Tripathi – Chief Product and Technology Officer at Skytap